ALMAnaCH seminar: “Modelling the past: the use of digital text analysis techniques for historical research”
Salle C434 & online after signing up
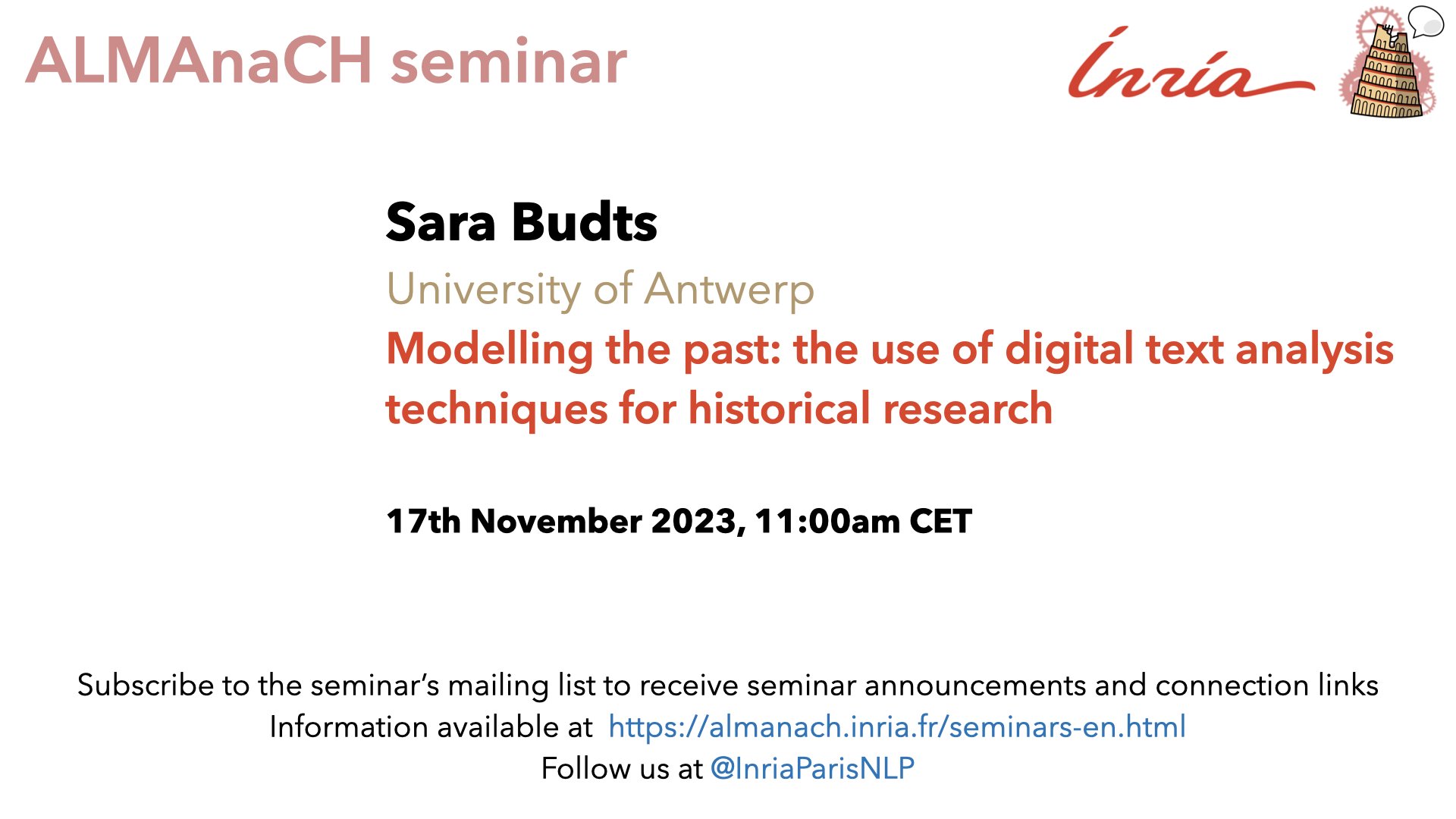
Speaker: Sara Budts, University of Antwerp
This seminar illustrates the benefits, caveats and shortcomings of the use of Natural Language Processing techniques to answer historical research questions by means of two recent projects that sit on the interface between the digital and the historical. The first project explores discursive patterns in lottery rhymes produced in the late medieval and early modern Low Countries, with a focus on the rhymes used by women. The lottery was a popular fundraising event in the Low Countries. Lottery rhymes, personal messages attached to the lottery tickets, provide a valuable source for historians. We collected more than 11,000 digitized short texts from five lotteries held from 1446 to 1606 and used GysBERT, a Language Model of historical Dutch, to identify distinctly male and female discourses in the lottery rhymes corpus. Although the model pointed us to some interesting patterns, it also showed that male and female lottery rhymes do not radically differ from each other. This is consistent with insights from premodern women’s history which stresses that women worked within societal, and in this case literary, conventions, sometimes subverting them, sometimes adapting them, sometimes adopting them unchanged. This research results from a collaboration with Marly Terwisscha van Scheltinga and Jeroen Puttevils. The second project is more practical in nature and addresses the design and implementation of a Named Entity Recognition (NER) system for the Johnson Letters, a correspondence of about 800 letters written by and to the English merchant John Johnson, all dated between 1542 and 1552. Due to the historical nature and relatively small size of the dataset, the letters required a tailored approach for NER-tagging. After manually annotating about 100 letters as ground truth, we set up experiments with Conditional Random Field (CRF) models as well as fine-tuned transformer-based models using bert-base-NER, hmBERT, and MacBERTh pre-trained language models. Results were compared across all model types. CRF models performed competitively, with combined sampling techniques proving effective for named entities with few training examples. bert-based-NER and hmBERT finetuned models performed better than MacBERTh models, despite the latter language model’s pre-raining with EModE data. This project was carried out in collaboration with MA-student Patrick Quick. Drawing on insights from these two projects, the talk will conclude with a brief discussion of the usefulness of NLP-methodologies for historical research more generally.