Short bio
Dr. Marc Lelarge is a researcher at INRIA. He is also a lecturer in deep learning at Ecole Polytechnique (Palaiseau, France) and Ecole Normale Superieure. He graduated from Ecole Polytechnique, qualified as an engineer at Ecole Nationale Superieure des Telecommunications (Paris) and received a PhD in Applied Mathematics from Ecole Polytechnique in 2005. Dr. Marc Lelarge received the NetGCoop 2011 Best Paper Award with his PhD student E. Coupechoux, was awarded the 2012 SIGMETRICS rising star researcher award and the 2015 Best Publication in Applied Probability Award with Mohsen Bayati and Andrea Montanari for their work on compressed sensing.
Topics of interest
Machine learning, deep learning, graphs and data analytics
Project in Prairie
Marc Lelarge’s research focuses on representation learning with an emphasis on explainability. Marc Lelarge develops algorithms for learning and mining from relational and graphical data and pursue collaborations in medical informatics (active learning and NLP for electronic health records). Marc Lelarge is teaching courses on deep learning at École Normale Supérieure and École Polytechnique.
Quote
Many scientific fields study data with an underlying graph or manifold structure such as social networks, sensor networks, biomedical knowledge graphs. The need for new optimization methods and neural network architectures that can accommodate these relational and non-Euclidean structures is becoming increasingly clear. Indeed, the statistical properties of data defined on high-dimensional graphs are very different form the stationarity, locality and compositionality assumptions at the core of the success of deep learning models in vision or NLP. For such type of data, I am working on a recent deep learning approach: graph neural networks able to learn a message passing algorithm and aggregation procedure to compute an embedding of the graph and its nodes.
Team
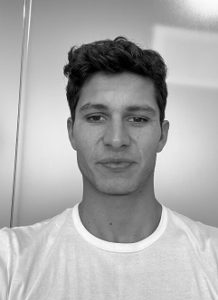
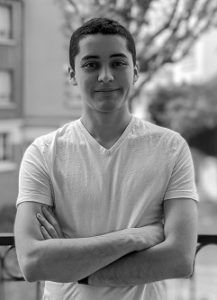
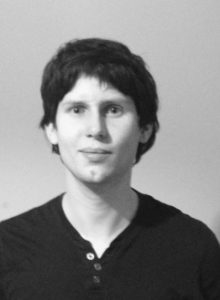