Catégorie offres: Closed
Call for Junior Group Leaders in Artificial Intelligence for Genetics, Biology & Medicine
Located in the heart of Paris on the Necker – Enfants Malades Hospital campus, Institut Imagine and Institut Necker Enfants Malades (INEM) are equipped with cutting-edge core facilities and foster a collaborative environment, uniquely positioning them to bridge research and patient care.
Institut Imagine and INEM seek outstanding Artificial Intelligence (AI) experts to join their teams as tenure-track Junior Group Leaders. These positions focus on applying AI to Biomedical Research, with a strong interest in Genetic Medicine, and offer internationally competitive start-up packages.
Successful candidates will develop an ambitious research program in collaboration with Institut Imagine, INEM, and the PR[AI]RIE AI community, pioneering novel AI approaches to understand, diagnose, and treat Mendelian and complex diseases.
Candidates should have a strong AI development background in at least one of the following areas:
• Imaging Analysis and Computer Vision
• Multimodal Data Integration
• Therapeutic Target Identification using machine learning and AI approaches
• Disease Pathogenesis Modeling by constructing AI-enhanced models or simulations
• Diagnosis and Treatment Response Modeling
.
Minimum Job Requirements:
● Ph.D. in Computer Science, Mathematics, Statistics, Data Science, Computational Biology, or a related field.
● Expertise in the theoretical underpinnings, implementation, and biomedical applications of AI methods, as evidenced by peer-reviewed publications.
Applications should be submitted to teamcallapplication@institutimagine.org and must include:
● A full CV (including a detailed list of publications)
● Past and current research interests (2 pages)
● Future research proposal (3 pages)
● Recommendation letters (3).
Applications must be received by March 8th 2025.
Pre-selected candidates will be notified from March 8th 2025 for auditions by the Scientific Advisory Board.
Integration of the new group can be effective from September onwards
Call for a Junior Group Leader in Systems and Computational Biology at Institut Imagine, Paris, France
Institut Imagine is a leading European research center dedicated to better understanding and treating genetic diseases. Imagine fosters a multidisciplinary and collaborative environment among 28 dynamic research groups by uniquely integrating basic and translational science.
Institut Imagine is seeking an outstanding Systems and Computational Biology expert to join our team as a Junior Group Leader. This position comes with an internationally competitive start-up package.
Key competencies:
● Established expertise in system biology, including skills in causal inference and statistical predictions and/or demonstrated experience developing mechanistic or dynamic models that mimic biological systems, as demonstrated by peer-reviewed publications.
● Ability to lead a system biology program, as evidenced by publications and grants
● Ability to collaborate with basic and clinical research teams to develop multidisciplinary project
Preferred Qualifications:
● Ph.D. and postdoctoral experience in a quantitative field such as Systems, Synthetic, or Computational Biology; Bioinformatics; Data Science; Statistics; Applied Mathematics; or a related discipline
● Experience with health applications of computational modeling and AI
● Experience with modern machine-learning libraries and tools, especially in bioinformatics
● Experience with cellular/molecular biophysics
.
Applications should be submitted to teamcallapplication@institutimagine.org and must include:
● A full CV (including a detailed list of publications)
● Past and current research interests (2 pages)
● Future research proposal (3 pages)
● Recommendation letters (3).
Applications must be received by March 8th 2025.
Pre-selected candidates will be notified from March 8th 2025 for auditions by the Scientific Advisory Board.
Integration of the new group can be effective from September onwards.
Computer Vision Internship at Avatar Medical
Paris, France + Partial Remote
https://avatarmedical.ai/
AVATAR MEDICAL is an exciting new MedTech start-up that radically enhances how surgeons
prepare their surgeries.
Based on technology developed at the Institut Pasteur and Institut Curie, AVATAR MEDICAL
uses advanced image processing algorithms coupled with extended reality (XR) to transform
medical images into lifelike patient avatars. This vehicle grants surgeons, patients, and students
an intuitive and powerful means of understanding the intricacies of medical imaging data.
AVATAR MEDICAL is a laureate of the prestigious BPI i-Lab, BPI i-Nov, and EIC Accelerator
competitions for innovative French startups and was the winner of the Laval Virtual 2022 Grand
Prix.
We are offering an exciting Computer Vision internship opportunity within our technology
Innovation Team. The successful candidate will work on a challenging project consisting in the
development of a mixed reality solution to train surgeons with specific surgical gestures. This is
a truly multidisciplinary project that combines medical imaging, image processing and
high-fidelity computer vision techniques for a distinct clinical training purpose.
Missions
● Work with surgeon partners to assess surgical training needs
● Assess different computer vision approaches for surgical use case
● Develop mixed reality surgical training prototype(s)
● Document the testing and development phases of said prototype
Required Skills
● Experience with computer vision approaches (e.g., OpenCV, SLAM, etc)
● Proficiency in C, C++ and Python programming languages
● Strong understanding of mathematics and linear algebra
● Confirmed problem-solving skills and ability to work well in a team
● Fluency in English (French language proficiency is not mandatory)
Nice to Have (not required, but a plus):
● Experience with Unity Engine and/or Unreal Engine
● Familiarity with AI object detection architectures (e.g., YOLO)
● Understanding of object-oriented design and SOLID principles
Why Join Avatar Medical?
● 30+ team members representing 7 nationalities, with offices in Paris, Saint-Malo, and
California
● Collaborate with talented and passionate professionals from around the world
● Join a fast-growing company at the intersection of technology and healthcare
● A competitive internship compensation package above the legal minimum
● International work experience
● Meal vouchers worth €10 per day, with €6 covered by the company
● Flexible hybrid work policy
Contract
Duration: Internship 6 month, from January 2025
Parisante Campus 10 rue d’Oradour Sur Glane 75015 Paris
Contact
If you are interested and qualified for this opportunity, please contact jobs@avatarmedical.ai
with the following:
● CV
● Motivation Letter
PhD Candidate/Institute Pasteur – PhD proposal: An Olfactory Pathway to Consciousness for Severe Brain-Injured Patients
PhD proposal: An Olfactory Pathway to Consciousness for Severe Brain-Injured Patients
Introduction
Consciousness is characterised by the ability to awake and interact with our environment. This state of wakefulness promotes sensory perceptions, whether from outside (the environment) or inside (for example, memory). These perceptions are then processed and made conscious through the action of specialised neural networks called correlates of consciousness (NCCs; Mashour et al., Neuron 2020). Studying NCCs has led to multiple theories explaining their functioning, including the global neuronal workspace theory, developed by the Dehaene and Changeux group (2004) and inspired by Baars (1988), and the integrated information theory, proposed by Tononi (Boly et al., J. Neurosci. 2017), and inspired by Edelman (1989). These theories emphasise the crucial role of the prefrontal-parietal network, particularly
the anterior cingulate cortex and the dorsal prefrontal cortex. They also highlight the necessity of functional integrity in the circuits of wakefulness, including the basal ganglia (ventral tegmental area) and brainstem nuclei integrated into the ascending reticular activating system, involving ponto-mesencephalic neurons and the locus coeruleus (Koch et al., Nat Rev Neurosci, 2016).
Acute primary brain injuries, such as intraparenchymal hematomas, subarachnoid haemorrhages, ischemic strokes, and traumatic brain injuries, can seriously threaten the functionality of central neural networks and cause disorders of consciousness (TDC), ranging from transient coma to prolonged states of altered consciousness (non-responsive wakefulness state, minimally conscious state). These disorders reflect the severity of the initial brain impact (Maas et al., Lancet Neurol. 2017). Measuring the extent of neuronal damage and predicting the speed and degree of consciousness recovery represents a double challenge for science and medical practice (Maciel, Contin Lifelong Learn Neurol, 2021).
The severity of brain injuries often necessitates admission to an intensive care unit and in some cases, neurosurgical or neuroradiological intervention. The management of potential complications, such as intracranial hypertension or secondary complications (e.g., hyperthermia, infections, hemodynamic, hydro-electrolytic, and metabolic disturbances), frequently requires deep sedation. This is often necessary, even in patients with pre-existing TDC, to optimise brain metabolism and perfusion (Oddo et al., Crit Care. 2016). Deep sedation for several days or more, in cases of refractory intracranial hypertension, can mask changes in the neurological status of the patient, complicating neurological assessment and prognostication. However, critical decisions regarding the limitation or withdrawal of treatment are often made in this complex context (Lazaridis et al., Neurocrit Care, 2019).
The consequences of these decisions, affecting patients, their families, society, and the healthcare system, are significant, placing the evaluation of the prognosis of severely brain-injured patients at the heart of an important debate for public health involving scientific, medical, and ethical considerations.
Predicting the recovery of consciousness and wakefulness depends on our ability to evaluate consciousness-related neural networks’ anatomical and functional integrity (NCCs). This involves examining the brain’s ability to perceive and integrate, in a conscious manner, stimuli ranging from simple to complex, thus revealing the integrity of the circuits responsible for wakefulness and consciousness. A multimodal approach is ideal, combining clinical assessment, advanced imaging techniques such as a positron emission tomography and functional magnetic resonance imaging, and neurophysiological methods including electroencephalography (EEG) and event-related potentials. However, healthcare professionals often face a dilemma between the accuracy of these diagnostic tools and their immediate
availability, particularly in critically ill patients in intensive care units.
EEG is particularly appreciated for its non-invasiveness, the possibility of bedside performance, and availability in most intensive care units. Additionally, it offers high temporal and spatial resolution, enabling the precise evaluation of neural changes in the brain in response to external stimulation, or “EEG reactivity” (Admiraal et al., Neurology, 2020). Significant advances have been made in interpreting EEG signals in patients with chronic disorders of consciousness (TDC), starting with the introduction of complex mathematical analysis methods for signal processing (quantitative analysis, such as spectral analysis or connectivity) (Sitt et al., Brain J Neurol, 2014), and the development of more sophisticated sensory stimulation techniques (Arzi et al., Nature, 2020). EEG reactivity to verbal motor commands has been detected in comatose patients, a condition known as cognitive-motor dissociation, and this reactivity has been linked to better long-term cognitive and functional prognosis (Claassen et al., NEJM, 2019). However, the effectiveness of these quantitative analyses and their general applicability are largely dependent on the quality of the EEG recording, which may be compromised in an intensive care setting due to factors such as artefacts, a limited number of electrodes due to intracranial medical devices, or the effect of sedation. It is hypothesised that overcoming these obstacles by removing the abovementioned
constraints would lead to more reliable qualitative and quantitative analyses.
Furthermore, regarding stimulation paradigms, the nature of the stimulus plays a crucial role in determining the types and localisation of neural networks activated. The complexity of the stimulus, whether passive or active and the sensory modality used are key factors influencing the specific activation of neural networks. The addition of an emotional component to the stimulus, such as the use of a patient’s name instead of a simple sound, can activate additional neural networks (e.g., temporal and limbic circuits) associated with interoceptive mechanisms, which are unique to each individual (Holeckova et al., Brain Res, 2006).
The hypothesis is that it is possible to find better access to the consciousness of severely brain-damaged patients by using stimuli known for their strong emotional and memory associations: olfactory stimulation. An approach combining generative models and advanced statistical structure is proposed to develop a new method for probing altered states of consciousness through olfactory stimulation.
This project consists of three initiatives
- The generative approach: Develop a generative algorithm of synthetic EEGs and a statistical structure procedure to create a high-resolution EEG (32 to 64 electrodes), free of missing electrodes, artefacts, and the effects of sedatives, using advanced modelling techniques known for their better generalisation capabilities. A detailed statistical structuring of the data will be carried out, as the problem involves multiple null models that need to be considered to ensure the generative model is conditioned to these models. We will train the generative model progressively using EEGs from healthy subjects, sedated subjects without brain injuries, brain-injured subjects without sedation, and finally, EEGs from brain-injured subjects under sedation. We will also use high-resolution databases (32 to 64 electrodes) to learn the generation of high-resolution EEGs from clinical recordings with 12 or less in cases where electrodes are missing
- The olfactory stimulus: Develop and optimise the olfactory stimulus to understand its effects on patients
- Predicting the fate of patients: Use the developed generative model and the optimised olfactory stimulus to characterise the evolution of consciousness in brain-injured patients in collaboration with Eleonore Bouchereau, a medical chief resident, and her forthcoming thesis. This will include a clinical study with the ethical paperwork submitted before the project’s start. The objective is to follow a variety of patients admitted to the service of Professor Sharshar, a director of neurophysiology. Based on these “experimental” trajectories, we will use physical modelling and Bayesian probability to create a “physics” of the trajectory of patients in the latent space learned by the generative model. This “physics” will then be used to predict the medical fate of new patients. This will deliver not only predictive models but also trajectories, along with the probabilities of these trajectories, providing a complete tool for medical decision-making.
The medical environment beyond the laboratories of PM Lledo and JB Masson
Our project is fundamental and highly theoretical in its execution, but its applications in medicine are immediate. Patients are recruited from the departments of anesthesiology-intensive care, neurology, and neurophysiology (Pr Martine Gavaret) of the GHU-Psychiatry and Neurosciences in Paris. These departments are expert centres for managing and neuro-prognostication patients with severe acute brain injuries
Regarding EEG data from healthy subjects, we have access to a public database and our own database, supported by the GHU-Psychiatry and Neurosciences in Paris. For sedated subjects without brain injuries, we have a database of approximately 250 EEGs collected from patients undergoing cardiac surgery at the European Georges Pompidou Hospital (validation by CERAR and CNIL already obtained for data exploitation). We are building a clinical database of patients with 64 high-resolution electrode EEGs collected in the neurology department (Pr. Guillaume Turc) of the GHU-Psychiatry and Neurosciences in Paris for brain-injured subjects not under sedation. For sedated subjects, whether brain-injured or not, we have a database of 322 patients collected during a multicenter study (PRoReTro – NCT02395868) that we have conducted.
Applications in medicine beyond the project
The production of a synthetic EEG enables the extrapolation of a high-resolution EEG from a standard clinical recording of a few electrodes, which will have multiple applications, particularly in neurology and psychiatry. This could lead to the development and commercialisation of a startup (PM Lledo and JB Masson have already launched their startups) In addition to its prognostic value, synthetic EEG could contribute to a better understanding of new neural networks and mechanisms involved in consciousness, as well as cognitive and functional disorders. This could also help refine medical diagnoses with improved
spatial resolution (e.g., in epilepsy). Additionally, our methods for removing the effects of sedation from EEG signals could be developed for use in perioperative monitoring or for optimising the titration of sedation during anesthesia-intensive care management.
The candidate
The successful candidate for this fully funded proposal should have a strong background in either physics or applied mathematics, advanced computational skills and the willingness to work in a highly interdisciplinary environment on highly complex medical data. This PhD will be joined to the one of Eleonore Bouchereau an MD who was recently granted “a poste Acceuil Inserm”. Candidates should send their application to dbc-epi-recrutement@pasteur.fr.
References
Mashour GA, Roelfsema P, Changeux JP, Dehaene S. Conscious Processing and the Global Neuronal Workspace Hypothesis. Neuron. 2020 Mar 4;105(5):776-798. doi: 10.1016/j.neuron.2020.01.026. PMID: 32135090; PMCID: PMC8770991.
Sergent C, Dehaene S. Neural processes underlying conscious perception: experimental findings and a global neuronal workspace framework. J Physiol Paris. 2004 Jul-Nov;98(4-6):374-84. doi: 10.1016/j.jphysparis.2005.09.006. Epub 2005 Nov 15. PMID: 16293402.
Boly M, Massimini M, Tsuchiya N, Postle BR, Koch C, Tononi G. Are the Neural Correlates of Consciousness in the Front or in the Back of the Cerebral Cortex? Clinical and Neuroimaging Evidence. J Neurosci. 2017 Oct 4;37(40):9603-9613. doi: 10.1523/JNEUROSCI.3218-16.2017. PMID: 28978697; PMCID: PMC5628406.
Koch C, Massimini M, Boly M, Tononi G. Neural correlates of consciousness: progress and problems. Nat Rev Neurosci. mai 2016;17(5):307‐21
Maas AIR, Menon DK, Adelson PD, Andelic N, Bell MJ, Belli A, et al. Traumatic brain injury: integrated approaches to improve prevention, clinical care, and research. Lancet Neurol. 1 déc 2017;16(12):987‐1048
Maciel CB. Neurologic Outcome Prediction in the Intensive Care Unit. Contin Lifelong Learn Neurol. oct 2021;27(5):1405
Oddo M, Crippa IA, Mehta S, Menon D, Payen JF, Taccone FS, et al. Optimizing sedation in patients with acute brain injury. Crit Care. 2016;20:128.
Lazaridis C. Withdrawal of Life-Sustaining Treatments in Perceived Devastating Brain Injury: The Key Role of Uncertainty. Neurocrit Care. 1 févr 2019;30(1):33‐41
Admiraal MM, Horn J, Hofmeijer J, Hoedemaekers CWE, Kaam CR van, Keijzer HM, et al. EEG reactivity testing for prediction of good outcome in patients after cardiac arrest. Neurology. 11 août 2020;95(6):e653‐61
Sitt JD, King JR, El Karoui I, Rohaut B, Faugeras F, Gramfort A, et al. Large scale screening of neural signatures of consciousness in patients in a vegetative or minimally conscious state. Brain J Neurol. août 2014;137(Pt 8):2258‐70
Arzi A, Rozenkrantz L, Gorodisky L, Rozenkrantz D, Holtzman Y, Ravia A, et al. Olfactory sniffing signals consciousness in unresponsive patients with brain injuries. Nature. mai 2020;581(7809):428‐33
Claassen J, Doyle K, Matory A, Couch C, Burger KM, Velazquez A, et al. Detection of Brain Activation in Unresponsive Patients with Acute Brain Injury. N Engl J Med. 27 2019;380(26):2497‐505
Holeckova I, Fischer C, Giard MH, Delpuech C, Morlet D. Brain responses to a subject’s own name uttered by a familiar voice. Brain Res. 2006 Apr 12;1082(1):142-52. doi: 10.1016/j.brainres.2006.01.089. PMID: 16703673.
Ho J, Jain A, Abbeel P. Denoising Diffusion Probabilistic Models [Internet]. arXiv; 2020 [cité 21 déc 2023]. Disponible sur: http://arxiv.org/abs/2006.11239
Khader F, Mueller-Franzes G, Arasteh ST, Han T, Haarburger C, Schulze-Hagen M, et al. Medical Diffusion: Denoising Diffusion Probabilistic Models for 3D Medical Image Generation [Internet]. arXiv; 2023 [cité 21 déc 2023]. Disponible sur: http://arxiv.org/abs/2211.03364
Verdier H, Laurent F, Cassé A, Vestergaard CL, Specht CG, Masson JB. Simulation-based inference for non-parametric statistical comparison of biomolecule dynamics. PLoS Comput Biol. 2023 Feb 2;19(2):e1010088. doi: 10.1371/journal.pcbi.1010088. PMID: 36730436; PMCID: PMC9928078.
Post doc – IA embarquée inspirée des structures neuronales des insectes F/H
Post doc – IA embarquée inspirée des structures neuronales des insectes F/H
Joined postdoctoral position with Orange on using drosophila larva biological neural networks for ultra-edge inference applications.
https://orange.jobs/jobs/v3/offers/135084?lang=fr
recruitment will be done by orange, feel free to contact us to dbc-epi-recrutement at pasteur dot fr
Votre rôle
Votre rôle est d’effectuer un travail de Post doc sur l’IA embarquée inspirée des structures neuronales des insectes dans le cadre du projet ANR « From biological neural connectomes to embodied ultra-edge AI » (AAPG2023) qui a démarré en 2024.
Contexte global et problématique du sujet :
La prolifération d’équipements connectés (senseurs, caméras, …) et d’équipements mobiles, les enjeux énergétiques (e.g. batterie limitée, RSE), éthiques (e.g. confidentialité des données) ou techniques (e.g. latence) entraînent la nécessité d’embarquer les modèles d’IA (Edge AI) et de concevoir des modèles frugaux en énergie et en complexité calculatoire.
Bien qu’ayant des systèmes neuronaux (connectome) de petite taille, les insectes peuvent réaliser des tâches complexes (apprentissage, calculs, décision d’actions, …) avec des bilans énergétiques très faibles et très peu de données d’apprentissage.
Depuis peu, le connectome de certains insectes est connu en détail. Par exemple, le connectome complet de la larve de drosophile constitué de 3016 neurones et 548000 synapses est maintenant connu en détail. Des sous-circuits de ce connectome impliqués par exemple dans des tâches de décision ou de classification ont également été caractérisés. Le but du projet ANR est de tirer parti de ces récentes avancées en biologie pour concevoir de réseaux de neurones artificiels compacts et énergétiquement frugaux directement inspirés des structures et principes de fonctionnement découverts dans le connectomes d’insectes. Un accent sera mis lors du projet sur le développement et l’implémentation de SNN permettant la classification et la prédiction de séries temporelles.
Objectif scientifique :
L’objectif du travail de postdoc est de s’inspirer des structures neuronales des insectes pour produire des réseaux de neurones classiques (DNN) et spiking neural networks (SNN) qui soient à la fois hautement compressés et frugaux en énergie ainsi qu’en données d’apprentissage.
L’un des principaux verrous à lever est la formalisation d’une méthodologie permettant le transfert des connaissances et des résultats de biologie vers la réalisation de réseaux de neurones DNN et SNN. Vous réaliserez ce travail de formalisation en étroite collaboration avec les équipes de l’Institut Pasteur. Vous appliquerez ensuite cette méthodologie à la conception et à l’implémentation de réseaux de neurones DNN et SNN bio-inspirés et démontrerez la pertinence de l’approche par des tests approfondis de leurs performances (calculatoire et énergétique).
Votre profil
Compétences (scientifiques et techniques) et qualités personnelles exigées par le poste :
- Machine learning, IA et réseaux de neurones
- Maitrise de langages de développement (python, C++).
- Curiosité, dynamisme et appétence pour l’innovation et la recherche multi-disciplinaire.
- Appétence pour la biologie et les neurosciences.
- Très bonne capacité d’analyse et de synthèse.
Formation demandée:
- Doctorat d’informatique, de physique, de mathématiques ou de neurosciences avec spécialisation en machine learning, intelligence artificielle et réseaux de neurones.
- Le doctorat doit être obtenu depuis 2022.
Expériences souhaitées :
- Spiking neural networks.
- Connaissance en biologie, neurosciences, biomathématique.
Le plus de l’offre
- Vous serez intégré(e) à l’équipe Homelan/AISH d’Orange Labs Sophia Antipolis à la pointe de l’expertise sur les services d’IA pour la maison connectée (IA embarquée et fédérée, équipement connecté, home gateway, …) et ferez partie d’un écosystème de chercheurs, de data scientists et d’ingénieurs d’étude permettant la mise en œuvre concrète des résultats.
- Ce postdoc s’inscrit dans le cadre d’un projet ANR qui a débuté en janvier 2024 pour une durée de 3 ans 1/2. Il donnera lieu à une importante collaboration entre l’équipe Homelan/AISH d’Orange Lab Sophia Antipolis et deux équipes de l’Institut Pasteur hautement spécialisées dans l’analyse et la modélisation des structures neuronales des insectes.
- Le sujet est très innovant. Il se base sur des résultats récents en biologie et en neurosciences permettant une approche novatrice pour la conception de réseaux de spiking neurons compacts et énergétiquement économes.
- Postdoc de 1an renouvelable deux fois.
Entité
L’ambition de la Division Innovation est de porter plus loin l’innovation d’Orange et de renforcer son leadership technologique, en mobilisant nos capacités de recherche pour nourrir une innovation responsable au service de l’humain, éclairer les choix stratégiques du Groupe à long terme et influencer l’écosystème digital mondial. Nous formons les expert(e)s des technologies d’aujourd’hui et de demain et veillons à une amélioration continue de la performance de nos services et de notre efficacité. La division Innovation rassemble, dans le monde, 6000 salariés dédiés à la recherche et l’innovation dont 740 chercheurs. Porteurs d’une vision globale avec une grande diversité de profils (chercheurs, ingénieurs, designers, développeurs, data scientists, …), les femmes et les hommes de Innovation sont à l’écoute et au service des pays et des business units pour faire d’Orange un opérateur multiservices de confiance.
Vous serez intégré(e) au sein du département HomeLan Services (environ 160 personnes) de la direction Home Services d’Orange Innovation. Ce département est composé d’ingénieurs, de chercheurs, de doctorants et d’experts techniques et assure la recherche, l’anticipation et le développement du groupe Orange, dans tous les domaines proches de la maison connectée, des réseaux domestiques, de l’efficacité et la sobriété énergétique, du ‘device management’ et de l’intelligence artificielle, dans le but d’offrir des produits et services à la pointe de l’innovation.
Contrat
Post Doc
M1/M2 internship: Developing Genotype-Conditioned Artificial Drosophila Larvae Behavioural Data Through Bayesian Program Synthesis
M1/M2 internship: Developing Genotype-Conditioned Artificial Drosophila Larvae Behavioural Data Through Bayesian Program Synthesis
Drosophila larvae have emerged as an ideal platform for simultaneously probing behaviour and the underlying neuronal computation1–5. Modern genetic tools allow efficient activation or silencing of individual and small groups of neurons. Combining these techniques with standardised stimuli over thousands of individuals makes it possible to relate neurons to behaviour causally However, extracting these relationships from massive and noisy recordings requires the development of new statistically robust approaches.
Recently, in experimental settings to probe defensive actions6 or understand the implementation of neuromodulation in small neural neworks7, larva populations exhibited significant deviations from usual behavioural features. These deviations lead to either the redefinition of features usually associated with these behaviours or to challenges in detecting them.
We aim to develop a Bayesian Program Synthesis8 (BPS) methodology for producing synthetic data that mirrors key characteristics found in experimental recordings. This method will entail an inference phase to identify large-scale parameters characteristic of larva populations. It will incorporate various generative models to either replicate the behavioural traits associated with particular genotypes or create bespoke behavioural features. Additionally, we plan to use a collection of small generative programs to create larva data under specific action conditions. The efficacy of this approach will be demonstrated by applying our behaviour analysis pipeline to the synthetic data and assessing its ability to facilitate transfer learning with annotated behavioral datasets.
Fig 1) Example of artificial generated from a pre-defined larva genotype
References
5. Winding, M. et al. The connectome of an insect brain. Science 379, eadd9330 (2023).
Scientific or technical background required for work program
The successful intern should have one of the following backgrounds:
- Statistical Physics, Applied Mathematics,
- Statistics & Bayesian Inference
Some fluency in Python is expected.
Contact: dbc-epi-recrutement at pasteur dot fr
M1/M2 Internship: Topology of neural connectomes
M1/M2 Internship: Topology of neural connectomes
Behavior and decision-making are determined by physical processes taking place in the complex environment of the brain. Experimental techniques have reached the point where it is now possible to map the complete wiring diagram (the anatomical connectome) of the brain of simple model organisms at the level of single synapses [1,2,3], and to control the activity of individual neurons in live animals and observe the resulting behavior [4]. Together this offers the occasion to reverse engineer the physical basis of behavior [4].
This project aims to employ computational, statistical, and machine learning methods to characterize the neural circuitry of the neuronal networks of small animals and investigate the influence of physical constraints on their topology. The project involves two subparts, which may be investigated individually or collectively as time allows and depending on the interests of the student:
- In search of biological neural network distances. Comparing networks is an open technical challenge as the multiplicity of existing tools does not bring a definitive answer [5]. For instance, comparing networks of different sizes is a non-trivial problem [5]. Network patterns — motifs [6]— are attractive structural features since their definition is a priori independent of the network size. We recently developed an inferential method that extracts statistically significant microcircuits from connectomes. This part of the project is focused on the comparison of circuit motifs as a proxy for network distances. The student would characterize and compare inferred sets of network motifs by exploring how dynamical models [7] are affected by motifs’ topological properties, e.g. their symmetry. Potential extensions of the subject would involve investigations of how a distribution of microcircuits affect the mesoscopic, or modular, structure of a network [8].
- Generative modeling of biologically-plausible brain networks from learnt latent embeddings. This axis aims to develop new generative network models based on embedding the nodes in a metric space where distances determine link probabilities based on a kernel that is learnt from data. This will involve generalizing latent space graph models [9] by learning the distance kernels defining the connection probabilities between neurons from data and possibly extending them to non-euclidean embedding spaces. In a second time, we aim to link these embeddings to higher-order circuit motifs and to the real 3D space embedding of neurons and other biophysical constraints acting on the neural network.
The internship may lead to a PhD scholarship in the lab to continue the project.
References
- [1] Winding et al., “The connectome of an insect brain.” Science 379: eadd9330 (2023).
- [2] Witvliet et al., “Connectomes across development reveal principles of brain maturation.” Nature 596: 257–261 (2021).
- [3] Dorkenwald et al., “Neuronal wiring diagram of an adult brain.” biorxiv https://doi.org/10.1101/2023.06.27.546656 (2023).
- [4] Jovanic et al., “Competitive Disinhibition Mediates Behavioral Choice and Sequences in Drosophila.” Cell 167: 858–870 (2016).
- [5] Hartle, H., Klein, B., McCabe, S., Daniels, A., St-Onge, G., Murphy, C., & Hébert-Dufresne, L. (2020). Network comparison and the within-ensemble graph distance. Proceedings of the Royal Society A, 476(2243), 20190744.
- [6] Milo, R., Shen-Orr, S., Itzkovitz, S., Kashtan, N., Chklovskii, D., & Alon, U. (2002). Network motifs: simple building blocks of complex networks. Science, 298(5594), 824-827.
- [7] Riascos, A. P. (2023). Dissimilarity between synchronization processes on networks. arXiv preprint arXiv:2309.13163.
- [8] Yamamoto, H., Spitzner, F. P., Takemuro, T., Buendía, V., Murota, H., Morante, C., … & Soriano, J. (2023). Modular architecture facilitates noise-driven control of synchrony in neuronal networks. Science advances, 9(34), eade1755.
- [9] Hoff et al., “Latent Space Approaches to Social Network Analysis.” JASA 1-00: 286–295 (2002).
Scientific or technical background required for work program
The successful intern should have training in one of the following fields: statistical or condensed matter physics, applied mathematics, or statistics.
Some fluency in Python and numerical simulations is expected.
Contact: dbc-epi-recrutement@pasteur.fr
Part-time postdoctoral research scientist position
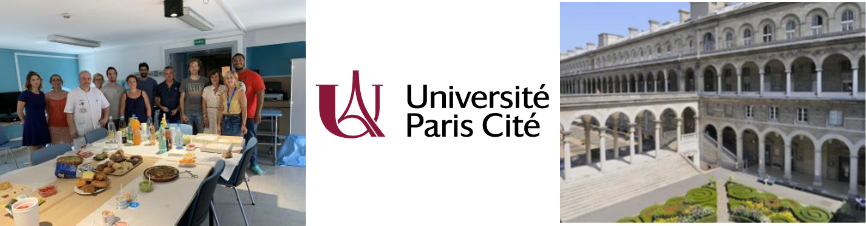
VIFASOM @Hôtel-Dieu-Paris
Pandore-IA project-Paris
- Type of contract: part-time (60%) fixed-term contract
- Level of qualification: PhD pr equivalent
- Function: postdoctoral research scientist
- Level of experience: recently graduated
- Start date: as soon as possible, before January 2024
The Pandore IA project
The objective of this project is to develop and validate a set of statistical learning methods and models
for predicting the physiological states of individuals performing intense physical activities under
specific conditions or environments, based on EEG, actigraphy, biological biomarkers, heart rate,
temperature, respiratory parameters…. It includes an experimental phase that will be carried out “in
the field” in an operational environment proposed by the VIFASOM team (Vigilance Fatigue and Sleep
Team in Hôtel-Dieu, and Bretigny), in relation with the French Army research institute IRBA) and
possibly with the French National sport institute (INSEP). To this end, a dedicated and sovereign
experimental environment consisting of a set of software tools, hardware and technical equipment
will be classified, stored, processed, exploited and data shared.
The two main domains of analysis would be:
- Detection of sleepiness and sleep states in operational situations and prediction of a staff’s state of consciousness (attention, awareness of the environment, interaction with the group and the environment, sleep, sleep disorders like insomnia, etc.)
- Prevention of hyperthermia with real-time prediction of exercise heat stroke and heat intolerance
This project aims to address the above issues by proposing a set of multidimensional AI methods
applied to physiological or other monitoring data.
The collaboration between the Université Paris Cité (VIFASOM) and SAAGIE (Rouen-France) company
will enable a team of researchers, engineers and medical and PhD doctors to participate in the design
and development of software modules and predictive models. Once developed, the team project will
deploy these prototype tools and test them in an ad-hoc technical environment in line with existing
research and expertise protocols.
Mission/Skills
The candidate will be involved in an interdisciplinary approach between neuroscience-ideally sleep and
vigilance-EEG, cognitive science, consciousness and computational approaches. The main missions will
be to program, run and analyze databases issues from research addressed by the teams involved in
the project on sleepiness- of athletes or subjects facing sleep restriction or operational environment.
The candidate needs to show high programming skills using Python, matlab, R or similar softwares.
Experience with human research on sleep, vigilance and thermoregulation is needed, as well as
redactional skills to write scientific papers and advancement reports
- Languages: Fluent English (speaking & writing)
- Relational skills: good interpersonal relation skills
- Other valued appreciated: sense of responsibility, self-driven, being curious, being team-playery
A regular basis physical presence will be expected for the term of the project. Working partly at home
may be discussed.
The supervising team
The medical and scientific supervision is organized with complementary skills for this interdisciplinary
project that associates human research data science and artificial intelligence with the supervision of
Damien Léger MD, PhD, VIFASOM, Hôtel-Dieu, Paris, Mounir Chennaoui, PhD VIFASOM IRBA, Paul
Bouchequet VIFASOM and Fabien SAUVET MD PhD, IRBA VIFASOM. Romain Picot-Clemente and
Patrick Giroux, from SAAGIE will provide scientific supervision for computational modelling,
development of software modules and predictive model
The work environment
The research team will be based at the VIFASOM lab of the Hôtel-Dieu, a beautiful hospital in the heart
of ancient neighborhoods of Paris, where participants will come for testing and treatment.
@Hotel-Dieu is also an exciting and innovative digital health data center supported by APHP, Université
Paris Cité and a group of health start up. The VIFASOM lab currently houses 3 PI and > 10 PhD students
and engineers working on various fields of cognitive science. This will offer the opportunity for fruitful
discussions and collaborations with SAAGIE in Rouen and the scientists in a stimulating workplace.
Nearby, the Université de Paris, Paris Public Health, PRAIRIE datascience and the Ecole Normale
Supérieure also offer many opportunities for exciting scientific training and conferences in cognitive science. The PhD students will have courses and scientific supervision at the Doctorate School Bio SPC
of Paris.
All supervisors endorse values of equity and diversity, and are committed to ensuring a safe,
welcoming, and inclusive workplace. Everyone is therefore strongly encouraged to apply.
Application
CV, motivational and recommandation letters should be sent to Pr Damien LEGER: damien.leger@aphp.fr Applications are reviewed on a rolling basis and all candidates will receive full consideration.
About
Université Paris Cité with its 61,000 students, 7,500 staff and 142 laboratories, is today the first
medical university in Europe and the University whose scientific publications are the most cited in
France.
The research team EA 7330 VIFASOM (Vigilance Fatigue and Sleep) was created in 2013. Co-directed
by Professor Damien LEGER and Mounir CHENNAOUI, it includes about thirty researchers who work on various themes related to sleep (Epidemiology, Effects of deprivation on alertness, performance,
risk of injury, and more recently on machine learning and IA).
Saagie, a company created in 2013, provides a platform to facilitate and accelerate the development
and deployment of projects dedicated to the exploitation and exploitation of big data (Big Data). This
solution integrates the best Big Data technologies of the moment around a Plug & Play orchestrator
supporting the DataOps methodology and allowing to embed artificial intelligence bricks to respond
to multiple use cases in fields of applications diverse and varied. Saagie now has a multidisciplinary
team of 90 people in Rouen, Paris, New York and London.
Ingénieur informatique Data-Challenge
Environnement et contexte de travail
PSL (Paris Sciences & Lettres) est une université globale qui fait dialoguer tous les domaines
du savoir et de la création en sciences, sciences économiques et sociales, humanités,
ingénierie et en arts. Elle inclue onze établissements, et notamment le Collège de France,
Dauphine – PSL et l’École normale supérieure (ENS)– PSL. Elle figure dans le top 50 des
meilleures universités mondiales (Shanghai, Times Higher Education, QS, CWUR).
Structures d’accueil : programme DATA de PSL et Centre de Sciences des Données ENS
La production et la collecte de données massives, alliées aux progrès des capacités de
stockage, d’analyse et de traitement, ont ouvert des perspectives scientifiques inédites
dans de nombreuses disciplines, comme par exemple les sciences du vivant, les sciences
cognitives, l’astrophysique, la sociologie quantitative.
Le programme transverse DATA fédère la formation de PSL en IA et aux interfaces des autres
disciplines scientifiques, ainsi qu’aux problématiques industrielles. Il se base sur la
plateforme web challengedata.ens.fr du Centre de Sciences des Données de l’ENS.
La plateforme challengedata.ens.fr propose des challenges de données pour l’enseignement
et la recherche, dans toutes les disciplines et à tous les niveaux, du doctorat jusqu’au
lycée. Les challenges proviennent d’entreprises, de services publics ou de laboratoires de
recherche. Ils sont utilisés dans des hackathons ou des cours du programme DATA de PSL,
mais aussi par d’autres universités, ainsi que l’éducation nationale pour des cours de lycées.
La plateforme valide les solutions proposées par les participants, en fournissant un score sur
des données de test. Elle offre aussi un environnement adapté à l’enseignement. Avec déjà
plus de 10 000 participants, elle est amenée à se développer considérablement en France et
à l’international.
Description du poste
L’objectif du poste est de développer et maintenir les fonctionnalités de la plateforme web
challengedata.ens.fr, des outils qu’elle met à disposition, et de participer à la sélection et la
validation de nouveaux challenges de données. Vous serez amené à prendre la responsabilité
informatique de la plateforme ainsi que ses axes de développement. Cela se fera en interface
avec l’équipe pédagogique et de beta testeurs des challenges, pour répondre aux besoins des
enseignements pluridisciplinaires en lien avec l’IA.
L’ingénieur(e) data challenges aura les missions suivantes.
- Maintenir et développer les fonctionnalités de la plateforme web challengedata.ens.fr
(Django). - Participer à la sélection et la validation de challenges de données proposés par des
entreprises, services publics ou des centres de recherche, au sein de l’équipe. - Développer des environnements Python pour l’enseignement de l’IA.
- Apporter un soutien informatique aux professeurs qui organisent les hackathons ou
les cours, en lien avec la plateforme.
Il s’agit d’un CDD sur 2 ans renouvelable.
Rattachement hiérarchique et fonctionnel
L’ingénieur(e) est placé(e) sous l’autorité hiérarchique du responsable du programme Data
de PSL et du responsable du programme ChallengeData de l’ENS.
Profil recherché
Diplôme et expérience professionnelle
• Niveau d’études minimum requis : Bac +4/5
• Domaine d’études : Informatique et/ou sciences des données/math. appliquées
• Niveau d’expérience minimum requis : débutant accepté
Savoirs et compétences attendus
La personne recrutée doit avoir de l’expérience en développement web avec Django et de
développement en Python. Le poste demande aussi un intérêt et une expérience de projets
en sciences de données en Python. Le/la candidate devra être autonome et proactive afin de
prendre en charge les axes de développement de la plateforme, en collaboration avec l’équipe
pédagogique ainsi que les bêta testeurs des challenges.
Compétences linguistiques
La maitrise de l’anglais indispensable.
Localisation
Sur site : Centre des Données de l’ENS, 45 rue d’Ulm, 75005 Paris et télétravail possible.
Candidater
CV et lettre de motivation à soumettre par email à stephane.mallat@ens.fr avec le sujet :
candidature ingénieur